Knowledge Distillation from ResNet to MobileNet for Accurate On-Device Face Recognition
DOI:
https://doi.org/10.5281/zenodo.15502091Keywords:
Artificial Intelligence, machine learning, biometric identificationAbstract
The developing of efficient facial recognition systems on low-resource devices requires models to optimize computational cost and performance for discrimination tasks. With this consideration, this research introduces a distillation process for distilling the rich representational power inherent in a high-achieving ResNet teacher model into a lightweight MobileNet student model, which is optimized for inference at a device level. With alignment between the feature embeddings of both student and teacher models, this solution significantly enhances MobileNet's recognition performance without incurring extra computational overheads.
Empirical evaluations on the CASIA‑WebFace dataset show that the distilled MobileNet achieves 95.7 % accuracy, 77.0 % recall, and an F1‑score of 86.9 %, closely approaching the ResNet teacher (97.2 % accuracy, 84.8 % recall) while shrinking the model size from 283.3 MB to 7.2 MB—a 39× compression. Compared with a conventionally trained MobileNet baseline (87.3 % accuracy, 62.8 % F1 at 14 MB), the distilled model delivers +8.4 % absolute accuracy and +24.1 % F1 improvements while halving the memory footprint. These results confirm that knowledge distillation can yield highly accurate yet resource‑efficient face recognition suitable for mobile and embedded applications.
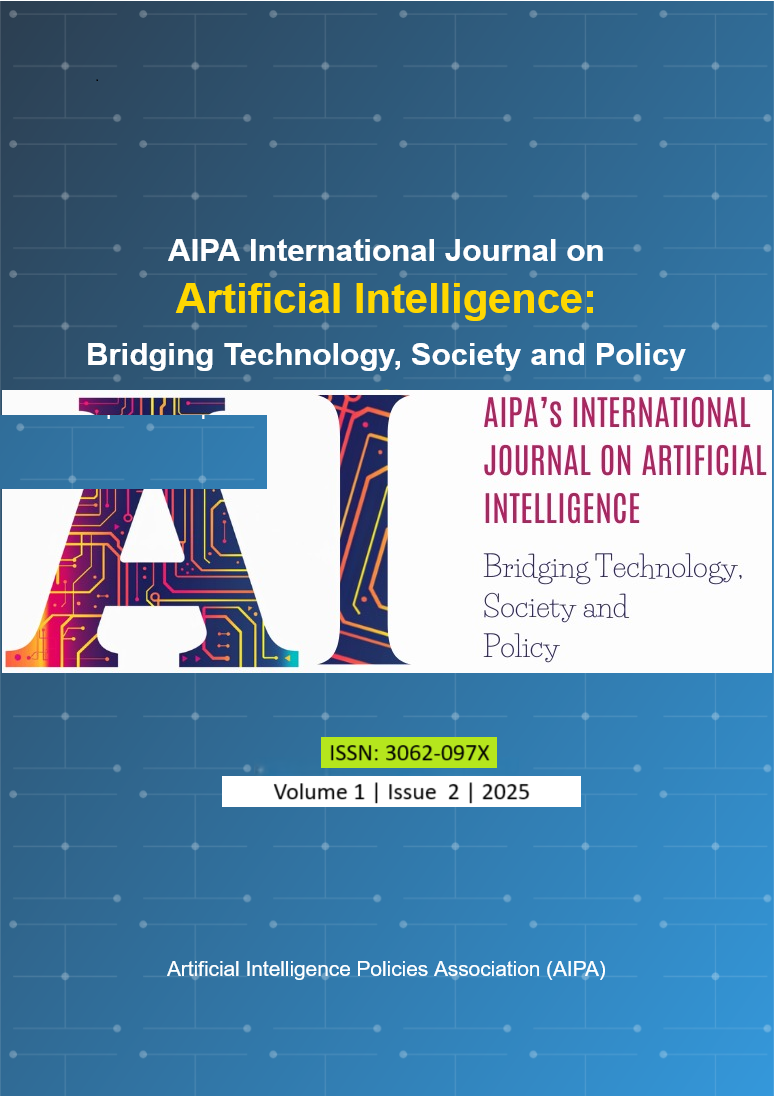
Downloads
Published
How to Cite
Issue
Section
License
Copyright (c) 2025 AIPA's International Journal on Artificial Intelligence: Bridging Technology, Society and Policy

This work is licensed under a Creative Commons Attribution 4.0 International License.